
Meet the Authors of the SUMITOMO ELECTRIC TECHNICAL REVIEW
We at Sumitomo Electric Group are driven to pursue technological innovations to create value for a better world.
Meet our experts and read their stories, where they share their experiences, hardships, and inspirations in their R&D endeavors.
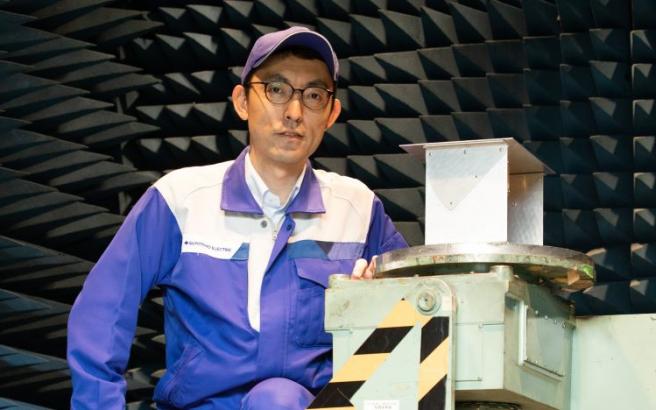
Yutaro Miki
Assistant General Manager,
AutoNetworks Technologies, Ltd.
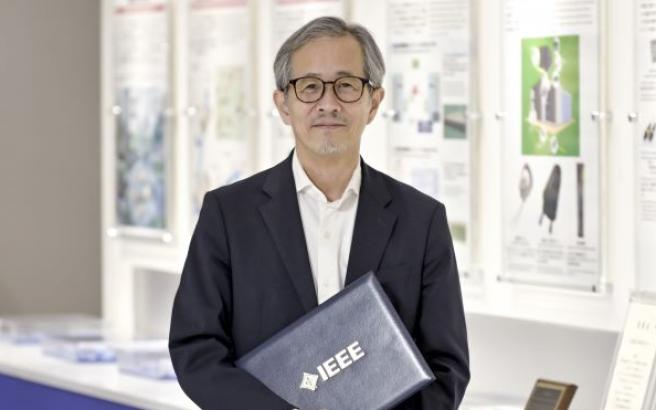
Masayuki Shigematsu
Chief Engineer, New Business Marketing & Promotion Div. and R & D Unit,
Senior General Assistant Manager, Human Resources Development Dept.
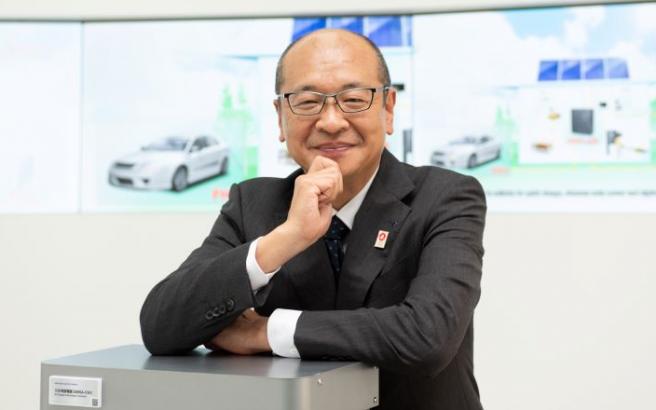
Hitoshi Hirata
General Manager,
Systems & Electronics Division
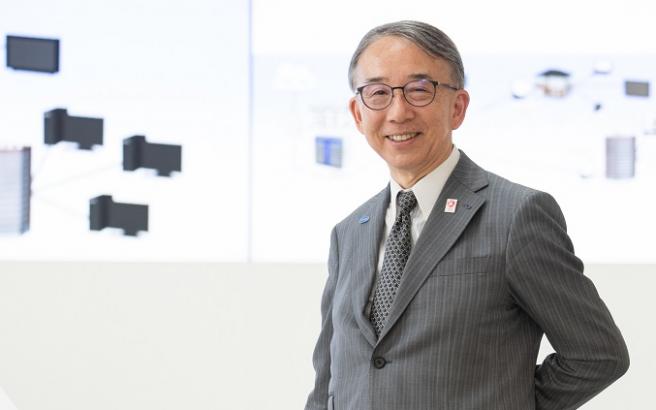
Hiroaki Nishimoto
Fellow,
Specially Appointed Management,
Chief Technology Supervisor, Infocommunications Business Unit & Innovative Network Solution Dept.
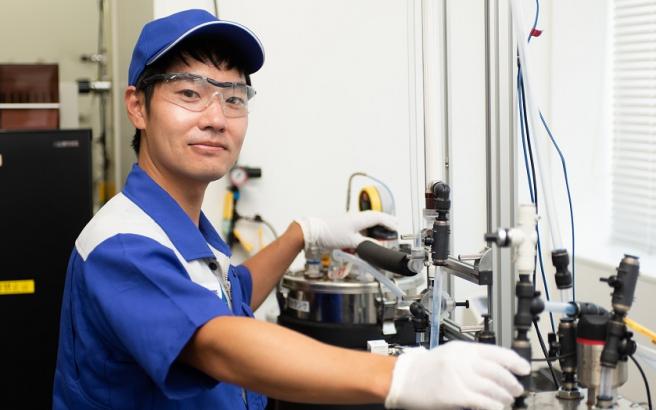
Hiroki Miyata
Assistant Manager,
Energy and Electronics Materials Laboratory
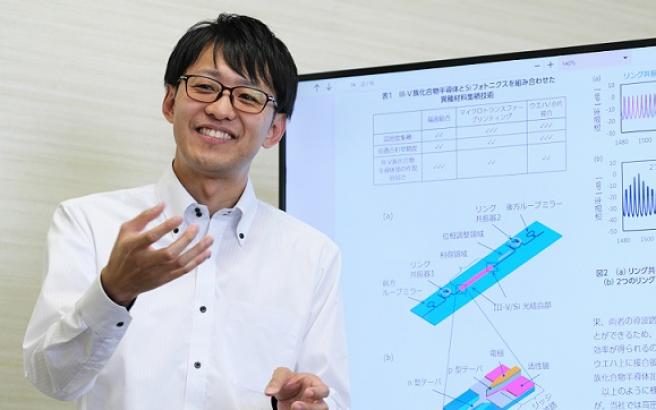
Dr. Takuo Hiratani
Assistant Manager,
Transmission Devices Laboratory
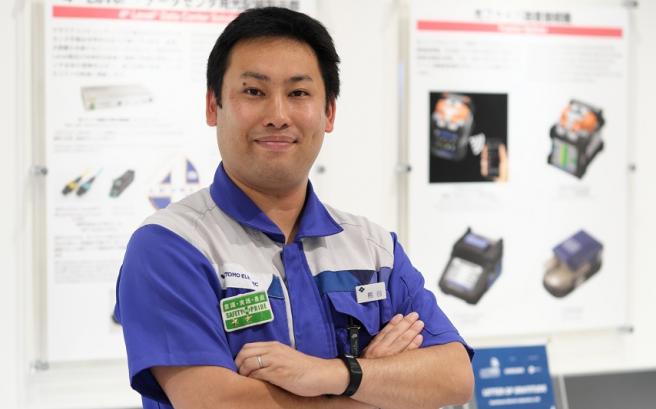
Dr. Tsutaru Kumagai
Assistant Manager,
Optical Communications Laboratory
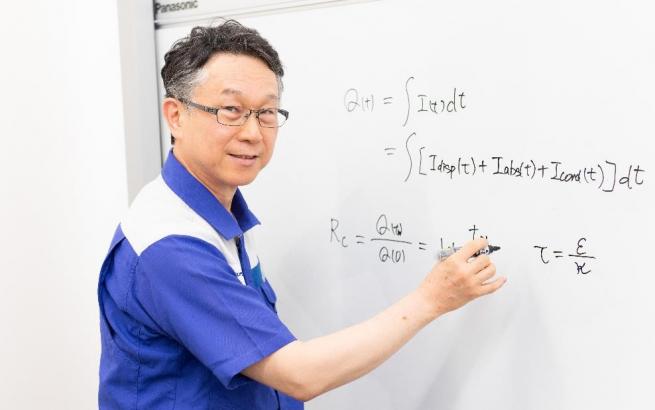
Yoitsu Sekiguchi
Assistant General Manager,
Energy and Electronics Materials Research Lab.
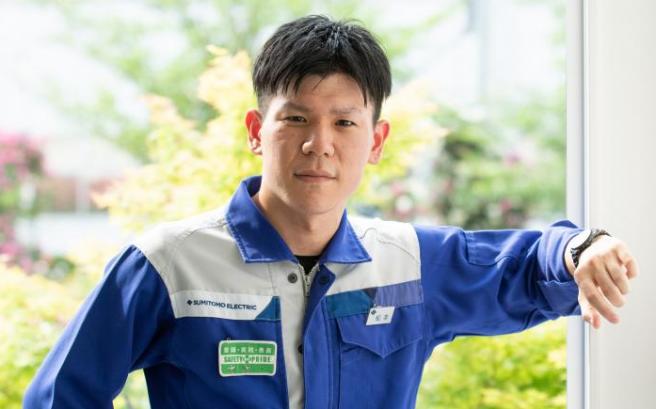
Shintaro Matsumoto
Information Network R&D Center
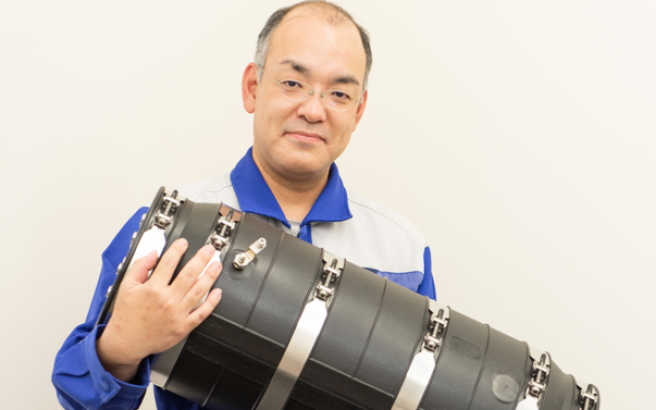
Tetsuo Goto
Assistant Manager,
Power Cable Projects Div.
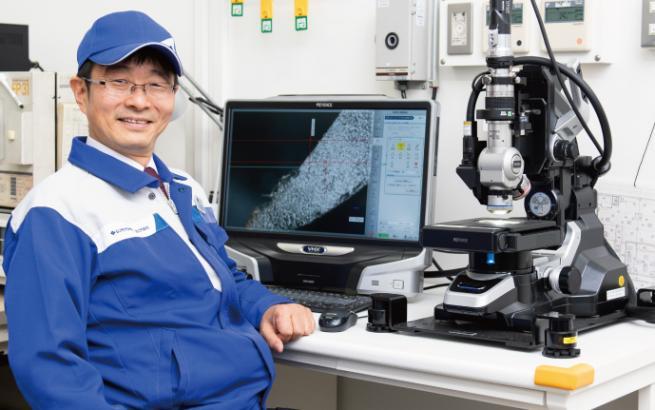
Soichiro Okubo
Assistant General Manager,
Frontier Technologies Lab.
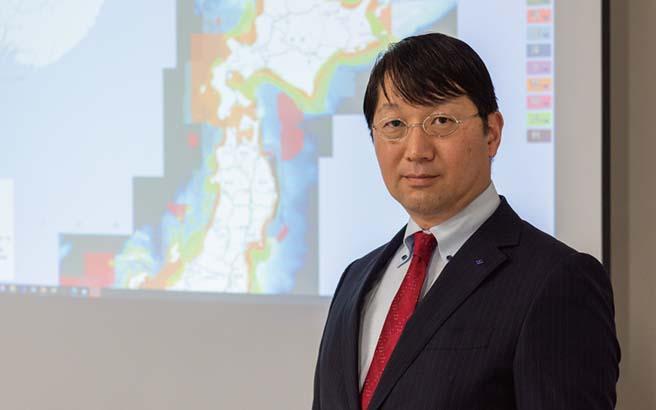
Shuji Mayama
Chief Engineer,
Power Cable Project Engineering Div.
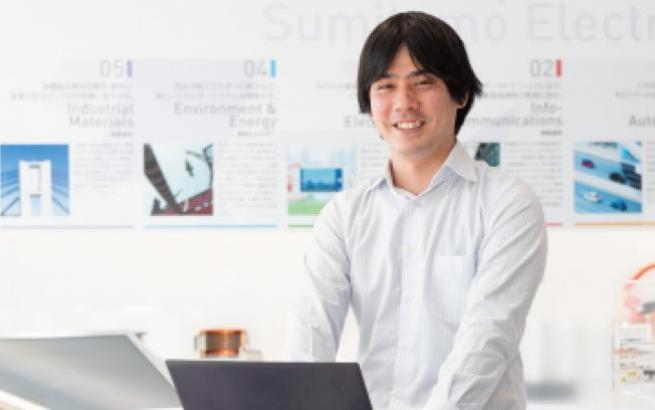
Kazuya Tokuda
Analysis Technology Research Center
SUMITOMO ELECTRIC TECHNICAL REVIEW
A journal of technical papers explaining the Sumitomo Electric’s technologies. Technical papers are available in PDF format.
Learn more