07 August 2023
Development of QTNN, a Spatiotemporal AI for Predicting Traffic Queue Length ーAchieved an Error of 40 m or Less in Predicting Traffic Queue Length One Hour Ahead in Tokyoー
-
Kyoto University
-
Japan Science and Technology Agency
-
Sumitomo Electric System Solutions Co., Ltd.
Overview
Traffic congestion not only causes daily stress but also results in a loss of approximately 10 trillion yen annually in Japan and contributes to the emission of greenhouse gases, making it a serious issue. As a technology to address this problem, AI that can predict when and where traffic congestion will occur is attracting attention worldwide. Assistant Professor Koh Takeuchi and Professor Hisashi Kashima of the Graduate School of Informatics at Kyoto University, together with Sumitomo Electric System Solutions Co., Ltd., have developed a novel spatiotemporal AI technology called "QTNN" (Queueing-theory-based Neural Network), which predicts the location and length of the queue of upcoming traffic congestion.
The most significant feature of QTNN is its ability to learn the relationship between changes in congestion and the road network based on knowledge from traffic engineering. In experiments predicting queue length one hour ahead on 1,098 road locations in Tokyo using data provided by the Metropolitan Police Department, QTNN achieved highly accurate predictions with an average error of 40 m or less. This result represents a remarkable 12.6% reduction in prediction errors compared to the state-of-the-art deep learning methods currently available.
Moving forward, the researcher group plans to conduct evaluation tests on certain roads to assess the reliability of this AI technology, as a step towards its full-scale implementation in real-world scenarios.
This achievement will be presented at the 29th ACM SIGKDD Conference on Knowledge Discovery and Data Mining, an international conference in the field of AI (machine learning and data mining), on August 6, 2023 U.S. West Coast time.
Illustration design: Yuka Tsuda (UMA / design farm)
Illustrator: Toshinori Yonemura
1. Background
The prediction of the length of future traffic queues holds great promise in society, as it can lead to smoother traffic and prevention of traffic congestion through proactive route guidance and signal control. The realization of an AI capable of precisely forecasting the presence and length of traffic congestion has been a difficult pursuit due to variations in the times of day, locations, and lengths, as well as its rapidly changing nature once it occurs. Moreover, for practical application of the AI, it is essential to establish its interpretability and reliability by clarifying the reasoning behind its queue length predictions. Therefore, the researcher group worked on the development of QTNN, a new spatiotemporal AI technology that achieves high precision and interpretability, by combining their expertise in traffic engineering based on years of field experience with the latest advances in deep learning and traffic big data, which have been attracting increasing attention in recent years.
2. Research methods and results
QTNN, a novel spatiotemporal AI technology proposed in this research, is built upon knowledge of traffic engineering and has the capability to learn the relationship between changes in traffic congestion and the road network through big data (Fig. 1). QTNN first predicts the average speed and traffic flow at each road segment using deep learning, based on data on congestion status at many road segments and the road network as a whole. It then forecasts the queue length while adjusting the traffic flow model commonly used in traffic engineering. By employing this two-stage prediction approach, the researcher group has realized queue length predictions that align with traffic engineering knowledge, even while leveraging state-of-the-art deep learning techniques.
This spatiotemporal AI technology was tested in an experiment predicting the queue length one hour ahead on 1,098 road locations within Tokyo over a span of two months using data provided by the Metropolitan Police Department (Fig. 2, Fig. 3). The results demonstrated a high precision prediction, achieving an average error of 40 meters or less by accurately anticipating both severe congestion and non-congestion cases (Fig. 4). This achievement represents a remarkable 12.6% reduction in prediction errors compared to the state-of-the-art deep learning methods currently available. Furthermore, QTNN utilizes traffic flow models, allowing for some interpretation of the prediction results, such as that represents the relationship between traffic flow, average speed, and queue length, "There will be a rapid increase in vehicle influx around 6:00, resulting in a significant increase in queue length," and "Queue length is reaching its peak, causing a decrease in traffic flow and average speed. Due to a certain traffic flow on surrounding roads, this queue will continue until around 10:00" (Fig. 5).
3. Ripple effects and future plans
QTNN is being considered for utilization in the Metropolitan Police Department's project aimed at enhancing traffic control systems through the utilization of AI and big data. Moving forward, the researcher group plans to conduct evaluation tests on certain roads to assess the reliability of this AI technology, as a step towards its full-scale implementation in real-world scenarios. The group also aims to realize a spatiotemporal AI technology that forms the foundation of urban infrastructure by flexibly utilizing information related to signal control, road construction, and accident occurrences to predict queue length.
4. About the research project
Part of this research was supported by the Japan Science and Technology Agency (JST) under the Strategic Basic Research Program PRESTO (Sakigake) in the Trustworthy AI area with the research code JPMJPR20C5.
In the Shin-Tosei project, a structural reform project by the Tokyo Metropolitan Government, it was conducted as a study related to a project for upgrading traffic control systems using AI and big data (https://shintosei.metro.tokyo.lg.jp/leading-project/leading-project-08/).
<Glossary>
◉ Route Guidance: Based on the traffic conditions of the road, the recommended route from the current location to the destination is calculated so as to avoid congested areas, and guidance is provided using car navigation systems or map applications on smartphones.
◉ Signal control: The allocation of green time for traffic signals and the timing of switching between green and red are adjusted based on the traffic conditions measured by vehicle detectors installed on the road.
◉ Queue length prediction AI: This is a technology that predicts queue length using traffic big data accumulated in cities. Research and development in this field are actively conducted worldwide. However, in many other countries, only average speed and traffic flow are mainly measured, which means there are very few studies that have focused on predicting queue length. The black box nature of internal calculations is considered another challenge in this area.
◉ Interpretability of AI: This term is used when expressing concerns about the reliability of AI due to its being a “black box” where it is not clear what computations are being performed internally, and the reasons behind its predictions are unknown.
◉ Traffic Engineering: A subfield of civil engineering that deals with research related to road traffic in general. Especially in recent years, research related to Intelligent Transport Systems has been gaining attention.
◉ Deep learning: A type of multi-layer neural network that learns patterns from vast amounts of data. It is a technology that drives the recent AI boom, however, it is also criticized for its black box nature, where internal computations are not transparent. In addition, unlike text, image, and voice analysis, the accuracy of traffic data analysis is still considered insufficient, even with deep learning.
◉Traffic big data: This term is used to refer to the vast amount of urban traffic data accumulated through car navigation systems, smartphones, and traffic control systems. In this research, it includes one year of data for average speed, traffic flow, and congestion length, measured every 5 minutes, at 1,098 locations on general roads within the 23 wards of Tokyo. Please note that the average length of road segments is 882 m, with a median of 750 m. In addition. Queue length was measured by means of measuring the length of a line of vehicles that repeatedly stopped and drove below a certain speed.
◉Spatiotemporal AI technology: This term is used to refer to AI developed to learn and analyze the complex spatiotemporal relationships of a phenomenon (such as the correlation between the temporal changes in traffic conditions and the spatial changes due to road connections) based on the information of when and where the data was measured.
◉Traffic model: This is a mathematical model used to describe traffic conditions. Typically, it describes the relationship between three variables: traffic flow, average speed, and density. However, traffic control systems cannot directly observe density. Therefore, QTNN uses a relationship model called the "sandglass model," which includes queue length.
◉Error: This is a value obtained by evaluating the degree of deviation between the predicted value and the actual observed value using the root mean squared error when predicting the future queue length up to a certain time ahead by using past traffic data.
<Researcher's comment>
In the fierce competition of AI research worldwide, we believe that Japan's expertise in precise data measurement technology and in-depth domain knowledge can provide a significant advantage over the rest of the world by combining them with cutting-edge spatiotemporal AI technology. We hope that this research will help envision new solutions to address traffic congestion problems and make a substantial contribution to the sustainability of cities. We will continue to pursue reliable and safe AI technology in the future.
<Paper title and authors>
Title | QTNet: Theory-based Queue Length Prediction for Urban Traffic |
Authors | Ryu Shirakami, Toshiya Kitahara, Koh Takeuchi, Hisashi Kashima |
Published in | Proceedings of the 29th ACM SIGKDD Conference on Knowledge Discovery and Data Mining (KDD '23) |
DOI | 10.1145/3580305.3599890 |
<Reference figures>
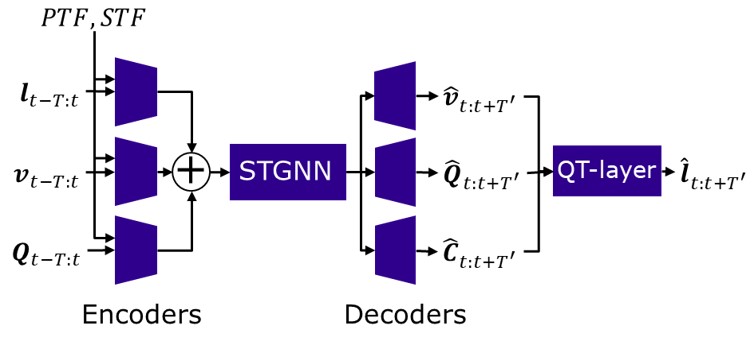
Fig. 1: Schematic diagram of QTNN. Using past queue length, average speed, and traffic flow data, the deep learning system (STGNN) predicts future average speed and traffic flow. While adjusting the traffic model based on these predictions (QT-layer), future queue length is predicted.
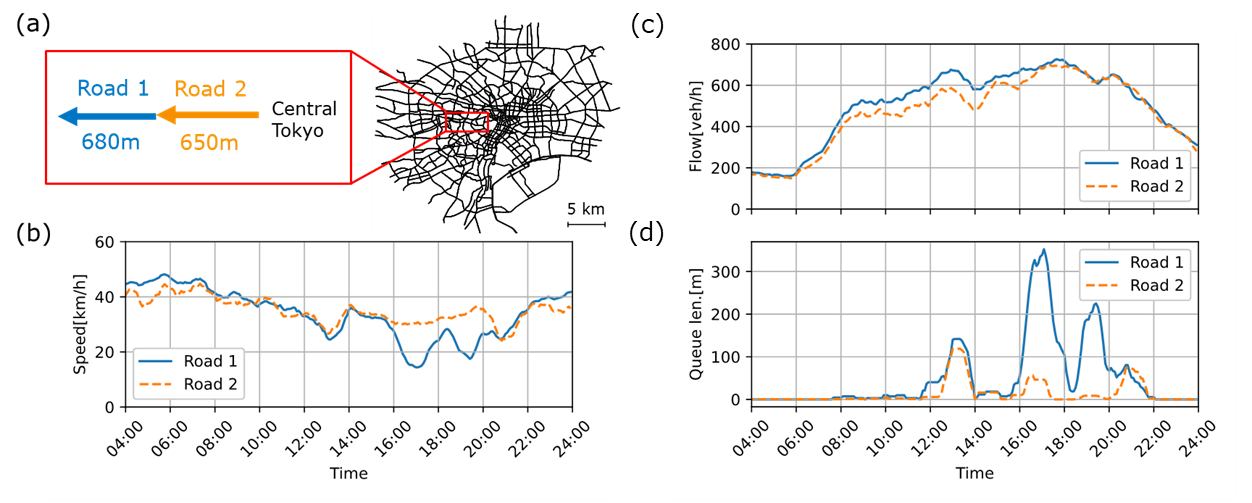
Fig. 2: (a) Example of the road network within Tokyo. (b), (c), (d) Examples of the temporal changes in traffic conditions on the two roads used in the experiment. When queue occurs in downstream section 1, it can be observed that queue subsequently arises in section 2.
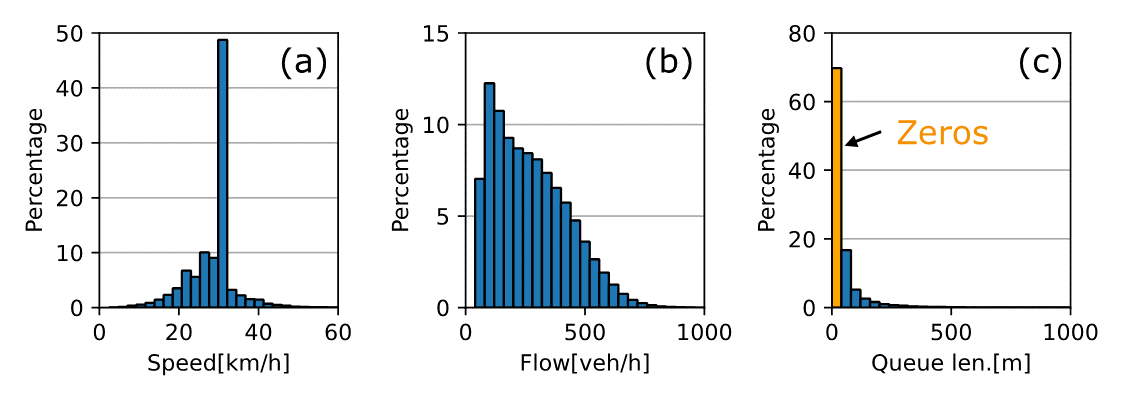
Fig. 3: Histograms of the data: (a) Average speed, (b) Traffic flow, and (c) Congestion length.
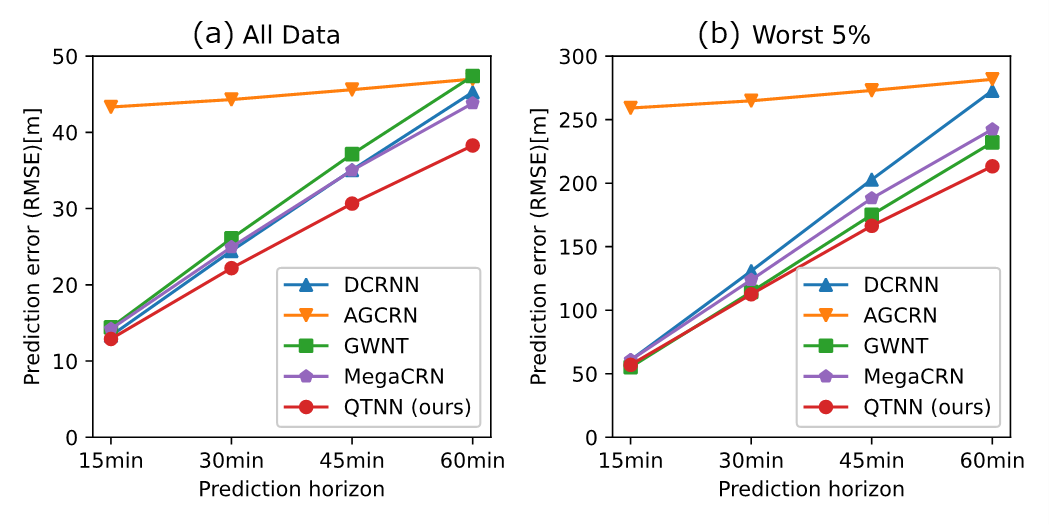
Fig. 4: The average prediction errors from 15 to 60 minutes ahead: (a) Evaluated with all data, (b) Evaluated with the most severe congestion data (top 5% of all data)
QTNN achieved lower errors than other state-of-the-art AI models (DCRNN, AGCRN, GWNT, MagaCRN) in all cases.
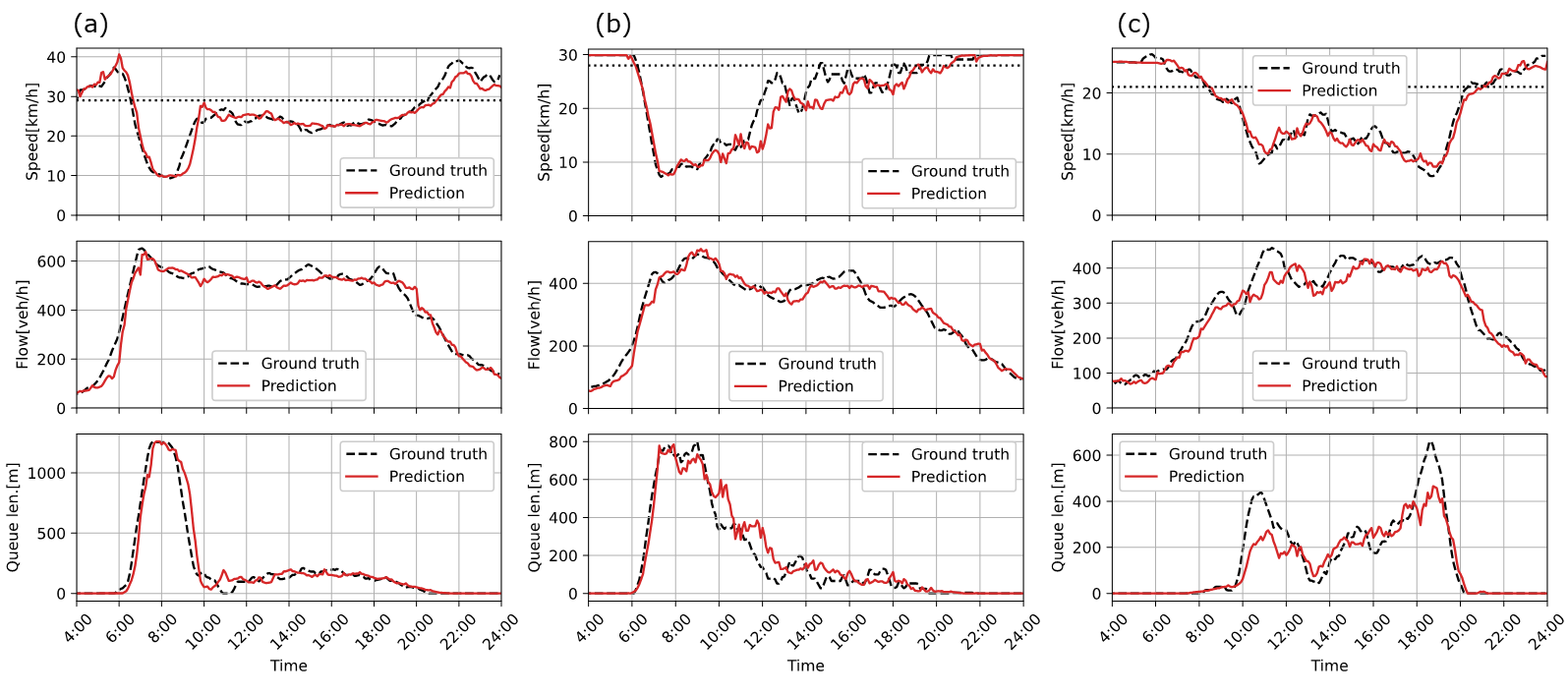
Fig. 5: Predicted results (red lines) of average speed, traffic flow, and queue length obtained by the proposed method compared to the observed values (dotted lines). It can be seen that the predictions by the proposed method are generally close to the observed values.